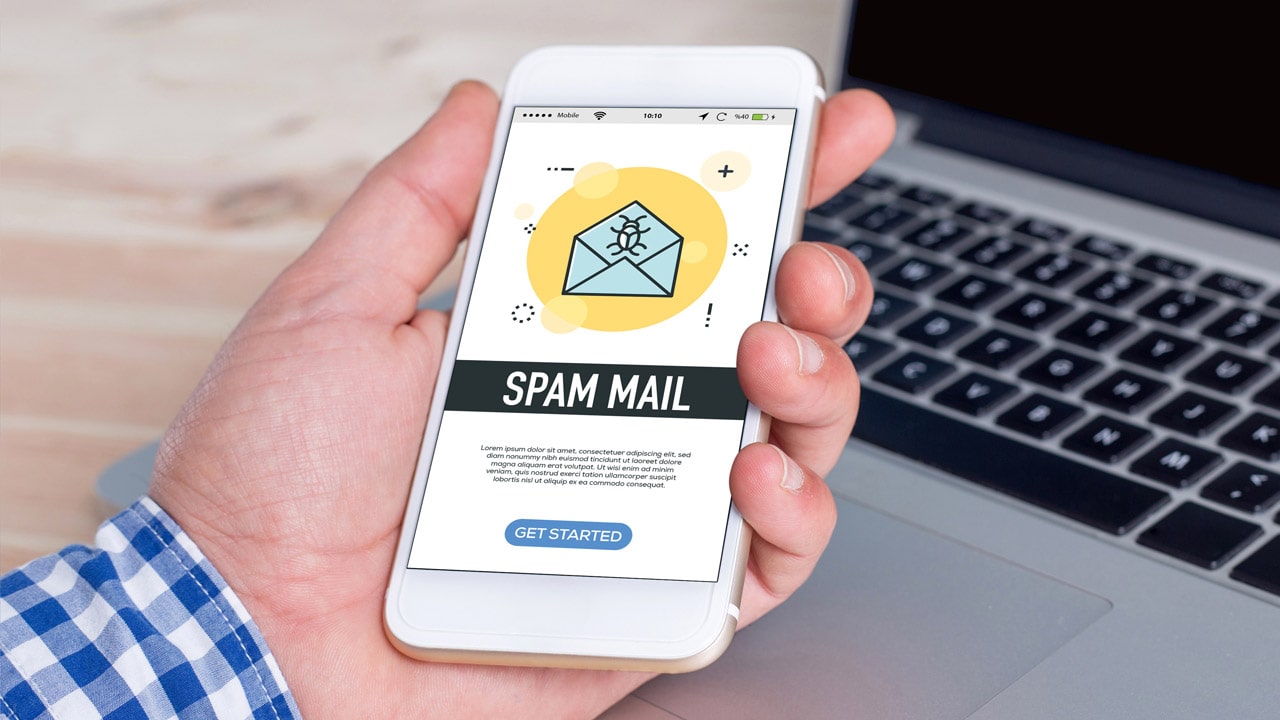
Project Summary
Enron Email Dataset
This dataset was collected and prepared by the CALO Project (A Cognitive Assistant that Learns and Organizes). It contains data from about 150 users, mostly senior management of Enron, organized into folders. The corpus contains a total of about 0.5M messages. This data was originally made public, and posted to the web, by the Federal Energy Regulatory Commission during its investigation. The email dataset was later purchased by Leslie Kaelbling at MIT, and turned out to have a number of integrity problems. A number of folks at SRI, notably Melinda Gervasio, worked hard to correct these problems, and it is thanks to them (not me) that the dataset is available. The dataset here does not include attachments, and some messages have been deleted “as part of a redaction effort due to requests from affected employees”. Invalid email addresses were converted to something of the form user@enron.com whenever possible (i.e., recipient is specified in some parse-able format like “Doe, John” or “Mary K. Smith”) and to no_address@enron.com when no recipient was specified.
Project Overview: Utilized Python to develop a machine learning model to classify emails as either “spam” or “ham” (not spam) using Python. The goal is to build an effective spam filter that can automatically detect and categorize spam emails to improve email management and reduce unwanted messages.
- DELIVERABLES
- Utilized Python to develop an advanced machine learning model to predict and classify spam emails with high accuracy and efficiency.
- Preprocessed a large dataset of emails, including both spam and non-spam messages, to ensure comprehensive training and testing of the model.
- Applied feature selection methods, including term frequency-inverse document frequency (TF-IDF) and word embeddings, to enhance the model’s ability to identify spam characteristics.
- Incorporated data imputation methods and handling of class imbalance to ensure model robustness and prevent overfitting, improving generalization to new data.
- Implemented a variety of machine learning algorithms, including Logistic Regression, and XGBoost, to determine the best-performing approach for spam detection.
- Trained and validated the model using cross-validation techniques, achieving high precision and recall rates while minimizing false positives and false negatives.
- Compared various models to arrive at a model with an accuracy of 97%.
- ANALYSIS IMPACT
- The spam email machine learning prediction system uses text preprocessing, feature extraction, and various classification algorithms to identify spam emails.
- By implementing and deploying this model, users can effectively filter out unwanted emails and improve their email management experience.